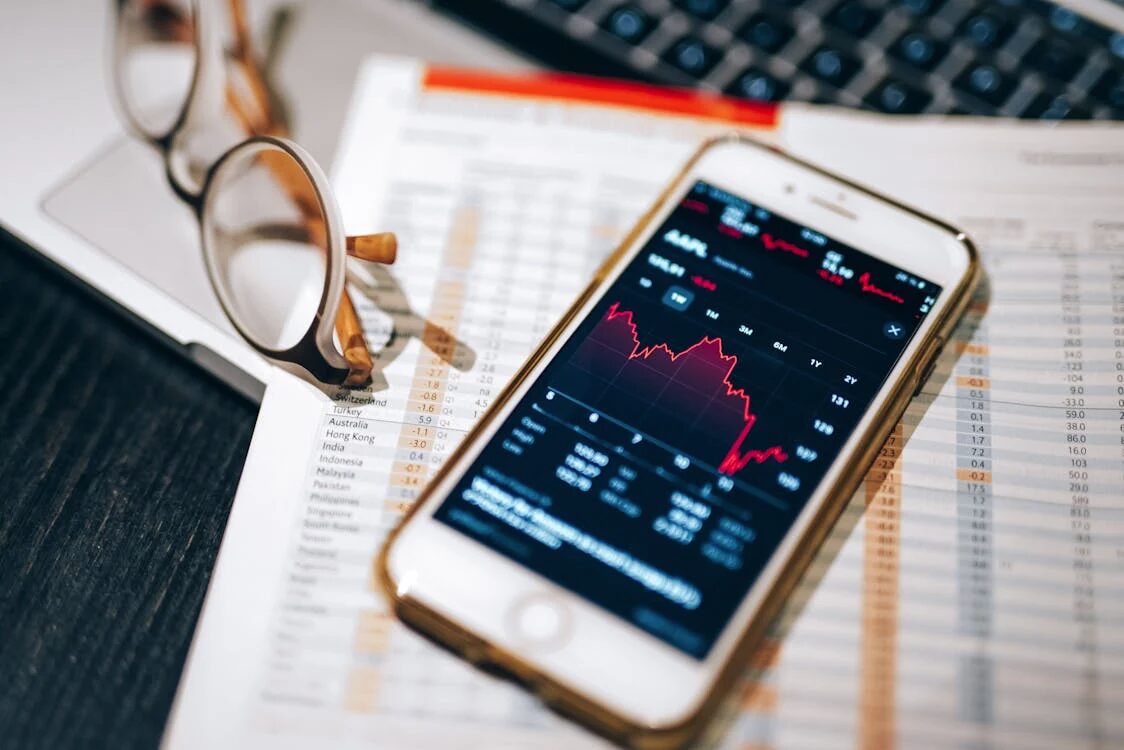
The rapidly expanding mobile app market demands more advanced analytical solutions beyond traditional options. Understanding your users better and boosting your app performance requires a Mobile App Analytics Platform that uses AI software to deliver more powerful and intelligent insights. That’s where artificial intelligence comes in.
The implementation of AI in mobile app analytics enables developers to analyze user behavior more accurately while forecasting trends and making informed decisions based on data. The results:
- Better user engagement
- Improved retention rates
- Higher conversion rates
- Faster problem detection
In this article we explore the transformative effects of AI on mobile app analytics and reveal the advantages of deploying these sophisticated technologies.
What you’ll discover:
- Why Traditional Mobile App Analytics Fall Short
- How AI Transforms Mobile App Analytics
- Key Benefits of AI-Powered Analytics
- Real-World Implementation Strategies
- Future Trends in AI Mobile Analytics
Why Traditional Mobile App Analytics Fall Short
Traditional mobile app analytics platforms deliver meaningful user interaction insights for your app. Their analysis measures app downloads along with active user statistics as well as session length and screen flow patterns.
But here’s the problem:
Traditional analytics tools function mainly in a descriptive mode that examines historical data. Traditional mobile app analytics platforms show you past user behavior but provide limited help to understand the underlying reasons for those behaviors or predict future actions.
The limitations of traditional analytics include:
- Data overload results from having excessive data but lacking clear actionable insights.
- These tools display problems only after they have already happened.
- Complex patterns need to be interpreted by human analysts which demands manual analysis.
- Limited personalization: One-size-fits-all approaches to user segments
Current app market conditions make these limitations crucial factors that determine whether an app succeeds completely or manages to survive.
If you’re still relying solely on traditional Mobile App Analytics Software without AI capabilities, you’re likely missing out on crucial insights that could dramatically improve your app’s performance.
How AI Transforms Mobile App Analytics
AI transforms mobile app analytics through intelligent automation across all stages of data analysis. We will now examine the precise steps of this transformation process.
AI-driven mobile app analytics platforms operate based on multiple critical technologies.
- These machine learning algorithms detect patterns and anomalies in user behavior which would be beyond human manual detection abilities
- Natural language processing technology allows for large-scale analysis of user reviews and feedback.
- Predictive analytics uses historical data to anticipate future user behavior patterns.
- Deep learning techniques reveal intricate connections between various metrics and user behavior patterns.
AI-powered analytics achieves transformation through its capability to analyze data beyond basic presentation. The system analyzes the data to detect significant patterns and then provides targeted recommendations. The integration makes analytics evolve from passive reporting mechanisms to active business intelligence frameworks.
Key Benefits of AI-Powered Analytics
Incorporating AI into your mobile app analytics stack unlocks numerous powerful advantages that enhance your app performance and business results.
1. Predictive User Behavior
AI provides insights beyond past user actions and predicts their future behavior. AI forecasts user actions by studying behavioral patterns.
- Which users are at risk of churning
- AI analytics helps identify which users show the highest potential to upgrade to a paid subscription.
- What features users will engage with next
- AI models can determine how updates to your application will influence user behavior patterns.
The ability to predict user behavior enables you to take proactive measures in both app development and marketing instead of reacting to events after they happen.
2. Advanced User Segmentation
Old-school analytics methods divide users according to basic demographic data and simple behavioral patterns. AI enables advanced segmentation through its ability to analyze complex behavior patterns, identify micro-segments with unique needs and behaviors, adjust segments automatically as user behavior changes and recommend personalized experiences for each segment.
- The AI system generates dynamic user segments by analyzing intricate behavioral patterns.
- Identifying micro-segments with specific needs or behaviors
- Automatically adjusting segments as user behavior evolves
- Recommending personalized experiences for each segment
The capability to segment and comprehend the large user base of 230 million AI app users by June 2024 remains extremely valuable.
3. Automated Anomaly Detection
AI has shown great effectiveness within mobile app analytics by detecting potential issues and anomalies before they escalate into major problems.
- Sudden drops in user engagement
- Unexpected changes in conversion rates
- Unusual patterns in user flow
- Performance issues affecting specific user segments
Artificial Intelligence systems keep track of these metrics round-the-clock and notify you about potential problems much faster than a human analyst would.
4. Personalized User Experiences
AI creates personalized user experiences through analysis of unique patterns and preferences for each individual.
- Custom content recommendations
- Personalized in-app messaging
- Adaptive user interfaces
- Tailored feature recommendations
Enhanced personalization leads to increased user engagement and retention which results in greater revenue generation.
Real-World Implementation Strategies
Are you prepared to integrate AI capabilities into your mobile app analytics system? This practical roadmap will guide you through the initial steps to start.
Start with Clear Objectives
Establish your goals before you start using AI analytics.
- Are you looking to improve user retention?
- Are you aiming to enhance your conversion funnel effectiveness?
- Your goal is to understand usage patterns to guide future development?
- Is predicting future user behavior necessary for your goals?
Defining your goals upfront will enable you to pick appropriate tools and concentrate your implementation work.
Choose the Right AI Analytics Platform
Different AI-powered analytics platforms offer varying levels of performance and capability. Look for solutions that offer:
- Integration with your existing analytics stack
- Scalability to handle your user base
- Specific AI capabilities that match your objectives
- User-friendly interfaces that make insights accessible
The United States generates 64% of the entire AI application revenue stream while the UK, Germany, Canada and China provide substantial contributions. The popularity of AI-integrated mobile applications paired with analytics services spans across worldwide markets.
Prepare Your Data
The quality of AI analysis depends entirely on the data it examines. Before implementation:
- Audit your current data collection practices
- Make sure every important event and user interaction is being monitored.
- Clean historical data to remove inaccuracies
- Implement consistent naming conventions
Start Small and Scale
Focus on implementing one or two AI capabilities rather than attempting to integrate every possible feature at once.
- Start with implementing AI for two or three specific use cases
- Validate results against traditional analytics
- Gather feedback from your team
- Develop your AI capabilities progressively by observing successful outcomes.
This method enables your team to learn and adapt without becoming overloaded.
Future Trends in AI Mobile Analytics
AI integration into mobile app analytics has only just begun to develop. These are the developments to expect in future AI mobile analytics.
Real-Time Personalization Engines
Next-generation AI analytics will progress from simple analysis toward immediate implementation of actions.
- Automatic adjustment of app experiences in real-time
- Dynamic pricing based on user behavior prediction
- Personalized content delivery without human intervention
- Interfaces which evolve according to user behaviors during application usage
Voice and Visual Analytics Integration
As interfaces evolve, so will analytics:
- Voice interaction analytics to understand conversational patterns
- The use of visual recognition techniques to study user interactions with AR/VR components
- Cross-device behavior analysis for seamless experiences
Key Takeaways
The use of artificial intelligence in mobile app analytics has shifted from being an optional feature to a critical component for successful apps. By implementing AI-powered analytics, you can:
- Understand your users on a deeper level
- Anticipate upcoming problems and stop them from impacting your business operations.
- Personalize experiences to drive engagement and retention
- Make data-driven decisions with confidence
Successful mobile applications will rely on intelligent analytics to drive every decision in addition to having excellent features. Remember that AI implementation is a journey. Establish clear objectives, select an appropriate platform and initiate focused use cases to achieve optimal results.